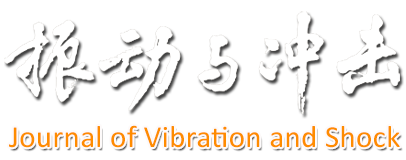

Investigation on Machine Condition Classification by Using Hilbert Spectrum Feature Extraction and Support Vector Machine
Hongkun Li Shuai Zhou Zhihui Sun
Journal of Vibration and Shock ›› 2009, Vol. 28 ›› Issue (6) : 131-134.
Investigation on Machine Condition Classification by Using Hilbert Spectrum Feature Extraction and Support Vector Machine
Hilbert time-frequency spectrum /
Feature extraction /
Gravity center /
Cyclostationarity /
Support Vector Machine /
{{custom_keyword}} /
/
〈 |
|
〉 |